A real world example:
Brewing beer is a touchy process that must balance the relationships between live cultures, bacteria, time, ambient and equipment temperatures, ingredients, equipment, elevation, and much more. The inherent variation can cause quality issues. One of the largest craft brewers in the US recently implemented machine learning (ML), artificial intelligence (AI) and historical process data to solve a batching problem that was causing a major quality issue and the loss of entire batches.
The brewmasters thought the problem was the relationship between pressure and temperature; instead it was an issue with the timing of batch processes determined by natural variances in yeast. They used ML/AI to build a model to alter the recipe and optimize batches on previously unknown relationships. By establishing a new process, the brewer eliminated lost batches associated with this quality issue and recaptured two weeks of extra capacity per lost batch.
So there are 11 axes of Quality 4.0 that can transform your business :
1. Data
Data-driven decisions have been at the heart of quality improvements for decades. Many recently updated standards re-emphasize the importance of evidence-based decision making. However, industry has a long way to go. As shown in the chart, much of the market continues to struggle with evidence while more mature companies have mastered traditional data and are now leveraging big data.
Systems gather three types of data: structured, unstructured, and semi-structured. Structured data is highly organized (CAPAs, quality events). Unstructured data is unorganized (e.g. semantics data, data from sensors and connected devices). Semi-structured data is unstructured and has had structure applied to it (e.g. metadata tags).
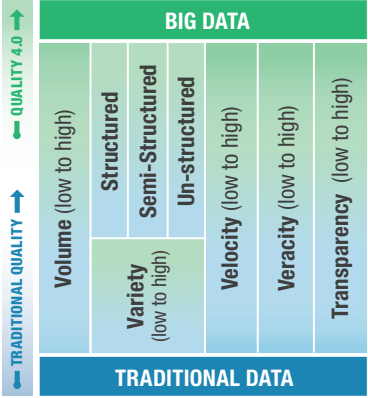
2. Analytics
Analytics unlock the insights captured within data. Analytics fall into four categories. Descriptive are the most traditional metrics to monitor known or suspected correlations. Quality generates descriptive metrics (number of open quality events), diagnostic metrics (quality process cycle times to identify bottlenecks), and predictive metrics such as trend analysis (application of trend rules to SPC data).
Companies can apply Big Data analytics, or ML/AI to traditional data or Big Data to determine correlations based on patterns in the data, which result in new insights. These insights are often much more specific than traditional analytics, such as predicting the failure of each specific machine. ML can identify, diagnose, and eventually predict a pattern that will result in a negative outcome such as product or process failure. Prescriptive analytics are more advanced; they predict failure and specify what should be done to address or change the outcome, and usually include some level of autonomous behavior. Descriptive, Diagnostic and Predictive analytics are performed in traditional quality with traditional data, but new insights are realized with ML/AI. Prescriptive analytics are exclusively in the ML/AI domain.
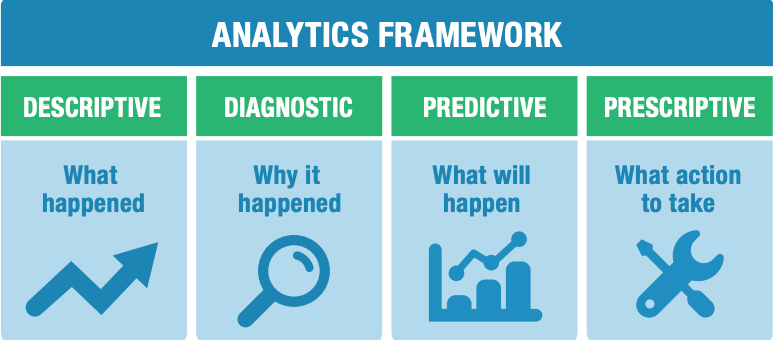
3. Connectivity
“Connectivity” is the connection between business information technology (IT) and operational technology (OT), where business technology includes the enterprise quality management system (EQMS), enterprise resource planning (ERP), and product lifecycle management (PLM), and OT is the technology used in laboratory, manufacturing, and service. Industry 4.0 transforms connectivity through a proliferation of inexpensive connected sensors that provide near real-time feedback from connected people, products and edge devices, and processes.
Connected people can leverage personal smart devices or intelligent wearable devices that sense workers. Connected worker initiatives typically have goals of increased efficiency and safety.
Connected products can provide feedback on their performance across their lifecycle. They can communicate use conditions, performance, and failure to perform.
Connected edge devices efficiently connect sensed equipment. This approach helps to avoid overburdening central OT systems by streaming large volume of sensor data. In this way, edge devices often perform analytics at the device, make predictive/prescriptive decisions (shut this machine down, and come for repair), and decide which data to send to central OT systems.
Connected processes provide feedback from connected people, products, and equipment into processes. Important applications of these concepts include Connected Products, Connected Laboratories, Connected Supply Chain, Connected Operations, and the Connected Worker.
4. Collaboration
Collaboration has changed dramatically in recent years, within and across companies and with customers. Social media has enabled the public to directly weigh in on quality, which has increased the visibility of quality issues. Manufacturers have started to leverage social listening to protect brands and learn new insights, which in turn fuels innovation.
Companies utilize digital messaging, automated workflows, and portals for traditional quality processes, but many have yet to fully embrace these technologies. Social media has significantly changed collaboration, enabling direct public input on quality issues, and manufacturers leverage social listening for brand protection and innovation.
Blockchain emerges as a transformative technology with potential for quality improvement in Supplier Quality Management (SQM) and traceability. It provides a secure, distributed ledger to track quality tests throughout the supply chain, addressing challenges like pass-through characteristics and traceability in recall scenarios.
5. App Development
App development means creating software applications that support quality management processes, including data collection, analytics and collaboration. As software has become more powerful, the trend has been to develop role-based apps for a better, simpler experience through multiple interfaces divided by role. Some examples are apps for wearables, augmented reality or virtual reality that will enhance the whole process of managing quality across the production line.As apps advance in terms of presenting relevant content to the user, where the user is, and in context with the world around him or her, they become increasingly powerful enablers of collaboration, competency, and efficiency.
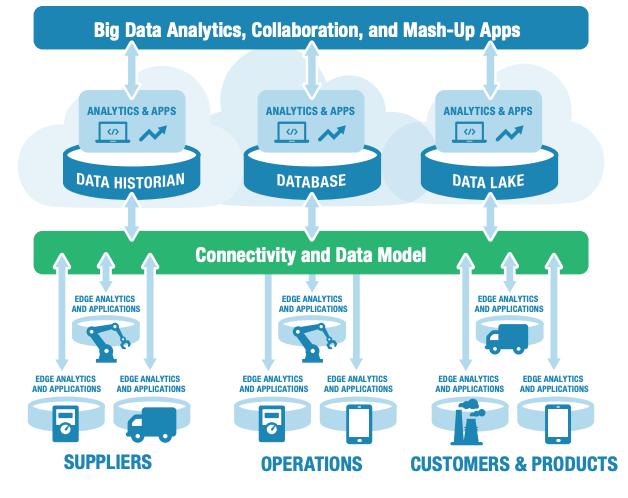
6. Scalability
Scalability is the ability to support data volume, users, devices, and analytics on a global scale. Cloud computing is an important contributor to scalability. Through Cloud, manufacturers can acquire Software as a Service (SaaS), easily adding capabilities and users, data, analytics, and devices without the need to acquire, install, and manage the software on premise. Data scalability is also important, particularly with connected devices. Data lake technologies have been developed to support the voluminous data sets inherent with connected devices and Big Data.
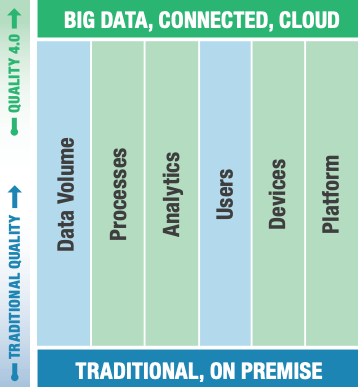
7. Management Systems
Management systems refer to technology-driven platforms that automate and streamline quality management activities, ensuring compliance, data accuracy and collaboration throughout the organization. The EQMS is the hub of quality management activities, providing a scalable solution to automate workflows, connect quality processes, provide centralized analytics, and foster collaboration within a common app. It is a hub because quality touches every part of the value chain and how it’s managed.
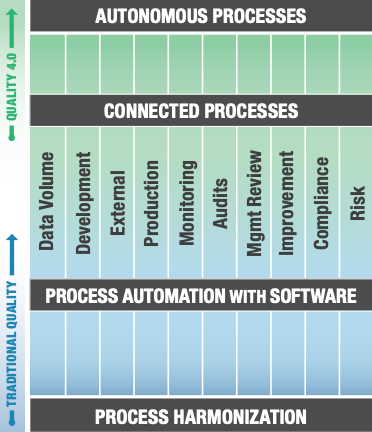
8. Compliance
Compliance using technology to ensure adherence to regulatory requirements, industry standards and internal policies are some of activities that we can improve in Quality 4.0. Life science manufacturers have a particularly heavy compliance burden. However, compliance is important to quality teams across industry since quality often takes a lead role in ensuring that processes, products, and services conform with requirements. Quality 4.0 introduces even more opportunities to automate compliance. Social collaboration provides a mechanism to share successful and failed approaches to compliance across groups, sites, and regions. Analytics can be used to alert organizations to potential compliance breaches or act to prevent the breaches. Integrated IT/ OT data models and/or collaboration technologies like blockchain can provide a data-driven approach that automates audit-ability.
9. Culture
Many leaders have an initiative to develop a culture of quality, since quality often owns process execution with insufficient participation and ownership from other functions. Culture means creating a work environment that values quality, encourages collaboration and empowers employees to take ownership of quality success. This is quite difficult to accomplish in traditional settings, in part due to regulatory burden, poor metrics and metric visibility, fragmented data systems and sources, and fragmented processes.
Quality can seem like a labyrinth to outsiders; more like a policing engine than a vehicle for improvement and performance.
10. Leadership
The quality function has an image and credibility gap with the rest of the organization. In part, this is because quality is seen as a department, as the quality police, and as a group with unclear alignment to corporate success. Quality improvement is clearly interesting to many, and quality is often a corporate value, but the work that the quality function does isn’t clearly connected to quality improvement or the corporate value by other functions and top management. Leaders from various departments recognize its potential and actively build initiatives around it, while supporting and leading efforts to ensure successful implementation. An organization with compliance-related objectives and initiatives will prioritize compliance-related practices, whereas an organization with performance initiatives will prioritize performance-related practices.
Objectives guide which initiatives receive attention and resources, and initiatives drive actions.
11. Competency
Competence is an individual’s ability to complete a task effectively and efficiently.Many have deployed structured approaches, which include Learning Management Systems to train competencies, assess competency through certification and Training Management, scale experience through centralized groups such as centers of practice/centers of excellence, and develop expertise.
On Quality 4.0 you can achieve that with:
Experience: Leverage social media to share experiences and lessons learned across internal groups, or even across industry
Expertise: Develop new expertise through learnings from ML/ AI. Leverage mashup apps and AR/VR to improve the expertise of workers.
Appraisal: Deploy connected worker strategies to sense worker actions, ensuring compliance, competency, efficiency, and safety.
Management: Encapsulate these learnings in Learning Management Systems, and provide VR experiences to improve training delivery
While competency may not be top of mind in Quality 4.0 initiatives, it could be the most powerful improvement resulting from Quality 4.0.
Conclusion
Quality 4.0 is a transformative approach to quality management that leverages digital technologies and data-driven insights. It emphasizes data-driven decision-making, connectivity and interoperability, smart and adaptive processes, and customer-centricity. Quality 4.0 enables real-time monitoring, proactive defect prevention, and continuous process optimization. It fosters collaboration and innovation by integrating social media, blockchain, and other advanced technologies. Quality 4.0 empowers industries to achieve higher product quality, operational efficiency, and customer satisfaction in a rapidly changing market.